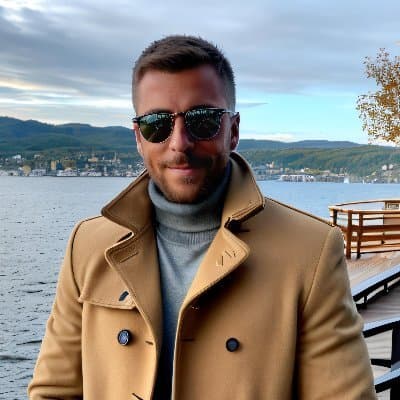
- Sep 4, 2023
- 2 min read
Machine Learning for eCommerce Solutions
Introduction
Machine learning is a branch of artificial intelligence that has garnered significant attention and rapidly evolved in recent years. With advancements in computing technology and the availability of vast amounts of data, machine learning algorithms are now able to learn and make predictions without being explicitly programmed. This groundbreaking approach is revolutionizing various industries, from finance to healthcare and transportation.
What is Machine Learning?
At its core, machine learning is a process that involves training a computer system to identify patterns and make decisions or predictions without being explicitly programmed. It enables the computer to learn from examples and experiences, continuously improving its performance over time. The algorithms used in machine learning analyze large datasets to discover patterns, correlations, and hidden insights that humans may not be able to discern.
Applications in Various Industries
Machine learning is being employed in numerous fields, reshaping the way businesses operate and providing new opportunities for innovation. In finance, for example, machine learning algorithms are used to predict market trends, detect fraud, and assess credit risks. In healthcare, machine learning algorithms analyze medical records to assist in diagnosing diseases, developing personalized treatment plans, and even predicting patient outcomes. Moreover, machine learning plays a vital role in the transportation industry, where it is used for autonomous vehicles, route optimization, and ride-sharing algorithms.
Machine Learning Techniques
Various machine learning techniques are utilized to accomplish different tasks. Supervised learning, for instance, involves training a model with labeled data to make accurate predictions for new, unseen data. Unsupervised learning, on the other hand, deals with analyzing unlabeled data to identify patterns and structure. Reinforcement learning, a more advanced technique, involves training an agent to make decisions based on rewards and punishments.
Conclusion
Machine learning has become a powerful tool that is transforming industries and uncovering new possibilities. By leveraging massive amounts of data and advanced algorithms, machines are now capable of learning and making decisions autonomously. This technology has the potential to enhance various processes, improve efficiency, and generate valuable insights. As machine learning continues to evolve, the possibilities are endless, and it will undoubtedly remain a crucial component of the future of artificial intelligence.
Machine Learning in eCommerce Solutions
Machine learning, a subset of artificial intelligence (AI), has revolutionized the eCommerce industry. As online businesses strive to deliver personalized shopping experiences and improve operational efficiency, machine learning algorithms provide invaluable tools to achieve these goals.
One of the primary applications of machine learning in eCommerce is in recommendation systems. By analyzing large volumes of customer data, machine learning algorithms can generate tailored product recommendations based on individual preferences and purchase history. This not only enhances the user experience but also increases the likelihood of conversion and repeat purchases, leading to higher sales and customer satisfaction.
Furthermore, machine learning algorithms can help eCommerce businesses automate various processes, from inventory management to pricing optimization. By leveraging historical data and analyzing market trends, algorithms can predict demand, optimize stock levels, and even suggest optimal pricing strategies to maximize profitability. This automation reduces human error and frees up resources, enabling businesses to focus on strategic decision-making and growth.
Another area where machine learning shines in eCommerce is fraud detection and prevention. Machine learning models trained on large datasets can quickly identify patterns and anomalies that indicate fraudulent activities, such as stolen credit card usage or account takeovers. By continuously refining these models, businesses can stay one step ahead of fraudsters and safeguard customer data and transactions.
In addition to improving day-to-day operations, machine learning can also play a significant role in enhancing customer service. Natural language processing (NLP) algorithms can interpret customer queries and respond in real-time, improving response times and satisfaction rates. Chatbots powered by machine learning can handle simple inquiries, provide product recommendations, and facilitate seamless transactions, all while offering a personalized touch.
The applications of machine learning in eCommerce are boundless. From supply chain optimization to personalized marketing campaigns, machine learning algorithms offer powerful tools to enable businesses to thrive in the evolving digital landscape. As technology advances, we can expect machine learning to continue shaping the eCommerce industry, revolutionizing the way we buy and sell products online.
In conclusion, machine learning holds immense potential for the eCommerce sector. By leveraging algorithms to generate personalized recommendations, optimize operations, detect fraud, and enhance customer service, online businesses can gain a competitive edge and deliver exceptional experiences to their customers. As the eCommerce landscape evolves, organizations that embrace machine learning will undoubtedly stand out in an ever-competitive market.
Machine Learning in Inventory Management Systems
Machine learning is revolutionizing the way businesses manage their inventory. By utilizing advanced algorithms and data analytics, machine learning enables businesses to optimize inventory planning, forecasting, and replenishment, leading to increased efficiency and reduced costs.
One key application of machine learning in inventory management systems is demand forecasting. Traditional methods rely on historical sales data and statistical models to predict future demand. However, these models often fail to capture complex patterns and fluctuations in consumer behavior. Machine learning algorithms, on the other hand, can analyze vast amounts of data from various sources, such as sales history, social media, and market trends, to generate more accurate demand forecasts. By understanding the underlying factors affecting demand, businesses can more efficiently allocate resources and minimize stockouts or excess inventory.
Another crucial aspect of inventory management is order optimization. Machine learning algorithms can analyze historical order data, supplier performance metrics, and external factors like seasonality or promotions to determine optimal order quantities and timings. This enables businesses to strike the right balance between maintaining sufficient inventory levels and minimizing carrying costs.
Additionally, machine learning can improve inventory replenishment strategies. By continuously monitoring inventory levels, sales patterns, and lead times, machine learning algorithms can predict the optimal time for reordering. This proactive approach ensures that the right products are available at the right time, reducing the likelihood of stockouts and improving customer satisfaction.
Moreover, machine learning algorithms can detect anomalies and identify potential risks in the supply chain. By analyzing data patterns, algorithms can flag unusual variations in demand, supplier performance, or delivery times, allowing businesses to take corrective actions and mitigate disruptions.
The integration of machine learning into inventory management systems also enables real-time decision-making. With the ability to process large volumes of data quickly, machine learning algorithms can provide businesses with up-to-date insights, allowing for faster responses to market changes or unexpected events.
In conclusion, machine learning has immense potential to enhance inventory management systems. Its ability to analyze vast amounts of data, generate accurate forecasts, optimize orders, predict replenishment needs, and identify risks makes it invaluable. By leveraging the power of machine learning, businesses can optimize their inventory management strategies, reduce costs, and gain a competitive edge in today's fast-paced market.
Machine Learning for Demand Forecasting
Demand forecasting is an essential aspect of business planning and strategy. Accurate predictions of future consumer demand enable organizations to optimize production levels, manage inventory efficiently, and make informed decisions when it comes to pricing and promotions. Traditional forecasting models rely on historical data and statistical analysis. However, with the advancement of machine learning, businesses are leveraging more powerful tools to achieve better forecasting accuracy.
Machine learning algorithms enable computers to learn from data and make predictions without being explicitly programmed. In the context of demand forecasting, machine learning models can analyze massive datasets that incorporate various factors such as historical sales, customer demographics, seasonality, promotions, and economic indicators. By capturing hidden patterns and trends in the data, machine learning algorithms can provide accurate demand forecasts.
One of the main benefits of using machine learning for demand forecasting is its ability to handle complex and nonlinear relationships between input variables and demand. Traditional forecasting models often assume linear relationships, which limits their accuracy. Machine learning algorithms, on the other hand, can identify intricate patterns and interactions that exist within the data, enabling them to capture more accurate demand patterns.
Another advantage of machine learning in demand forecasting is its adaptability to changing environments. Demand patterns can shift due to various factors such as market trends, competitor actions, or economic fluctuations. Machine learning models can adapt to these changes by continuously learning from new data and adjusting their predictions accordingly. This adaptability allows businesses to make more informed decisions and quickly respond to market dynamics.
Furthermore, machine learning models can integrate and analyze various data sources simultaneously. For instance, a demand forecasting model can incorporate not only historical sales data but also external data sources such as social media trends, weather patterns, or economic indicators. By considering multiple data sources, machine learning algorithms can improve demand forecasting accuracy and generate more holistic insights for decision-making.
In conclusion, machine learning offers significant advantages for demand forecasting. By leveraging complex algorithms, these models can capture nonlinear relationships, adapt to changing environments, and integrate multiple data sources. The improved forecasting accuracy enables businesses to optimize their operations, reduce costs, and make more informed decisions to meet consumer demand effectively. Implementing machine learning for demand forecasting can provide a competitive edge in today's rapidly evolving business landscape.
Conclusion
In conclusion, machine learning is a revolutionary technology that has the potential to transform countless industries and improve various aspects of our lives. Through its ability to extract insights and make accurate predictions from large amounts of data, it enables businesses to enhance their decision-making processes, optimize operations, and deliver personalized experiences to customers. Machine learning algorithms have already been successfully applied in diverse fields such as healthcare, finance, marketing, and transportation, yielding significant improvements and opening up new possibilities.
However, despite the immense potential of machine learning, there are several challenges that need to be addressed. The lack of transparency and interpretability of certain machine learning models raises concerns around ethics, privacy, and fairness. It becomes crucial for organizations to implement robust and ethical frameworks to ensure responsible use of machine learning algorithms and safeguard against bias or discrimination.
To fully embrace the benefits of machine learning, individuals and businesses should invest in acquiring the necessary skills and knowledge. Educating oneself on the fundamentals of machine learning and staying updated with the latest advancements will enable professionals to harness its power effectively. Organizations should also foster a culture of continuous learning and provide resources and training to their employees to unleash the potential of machine learning within their operations and strategies.
Looking ahead, machine learning will continue to evolve and disrupt various sectors. The ongoing advancements in artificial intelligence and technologies like deep learning promise even more powerful and sophisticated models, capable of addressing complex problems and delivering unprecedented accuracy. As the integration of machine learning becomes more widespread, collaboration and interdisciplinary work will play a crucial role in further driving innovation and pushing the limits of what is possible.
In conclusion, machine learning is transforming industries, enabling data-driven decision-making, and unlocking new opportunities. It has the potential to revolutionize our world, making processes more efficient, predictions more accurate, and experiences more personalized. As technology continues to progress, embracing machine learning and staying at the forefront of its advancements will be vital for individuals and businesses alike.
Are you ready to unlock the potential of machine learning? Stay curious, stay informed, and join the machine learning revolution today!