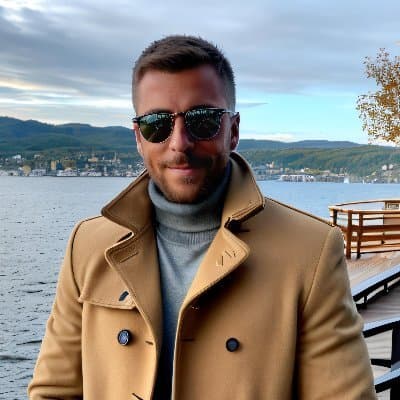
- Sep 4, 2023
- 2 min read
The Power of Pearson Correlation in Product Recommendation Engines
Pearson Correlation: Understanding the Measure of Linear Relationship
The Pearson correlation coefficient, often referred to as Pearson correlation or simply Pearson's r, is a statistical measure that quantifies the strength and direction of a linear relationship between two variables. It measures the extent to which a change in one variable corresponds to a change in another variable. This widely used statistic is invaluable in fields like economics, social sciences, and psychology, where researchers often seek to understand the association between two variables.
Named after its developer, Karl Pearson, the Pearson correlation is considered one of the foundational tools of statistical analysis. It is based on the principle that a linear relationship between variables can be described by a straight line. By calculating the correlation coefficient, researchers can determine whether two variables are positively, negatively, or not associated at all.
The Pearson correlation coefficient ranges from -1 to 1, with -1 indicating a perfect negative correlation, 1 representing a perfect positive correlation, and 0 denoting no linear correlation. A positive correlation means that as one variable increases, the other variable tends to increase as well. Conversely, a negative correlation suggests that as one variable increases, the other variable tends to decrease. It is important to note that correlation does not imply causation; it only measures the strength and direction of the linear relationship between the two variables.
To calculate the Pearson correlation coefficient, researchers use a precise formula that involves the covariance of the two variables and their standard deviations. By applying this formula, they can obtain a single value that represents the strength of the relationship between the two variables.
Understanding the Pearson correlation coefficient is essential for various data analysis tasks. It enables researchers to make informed decisions, identify patterns, and predict outcomes based on the relationship between variables. Additionally, it aids in the creation of predictive models and the evaluation of statistical significance.
In the upcoming articles, we will delve deeper into the concept of Pearson correlation, explore its applications, and discuss its limitations. With this foundational knowledge, you will be equipped to dive into the world of statistical analysis and uncover invaluable insights.
Why Pearson correlation is crucial in product recommendation engines
Pearson correlation is a statistical measure that evaluates the linear relationship between two variables. In the context of product recommendation engines, it plays a crucial role in predicting the preferences of users and suggesting items that they may like. This method is widely used in the field of data science and machine learning for generating accurate and personalized recommendations.
One of the main reasons why Pearson correlation is so essential in product recommendation engines is its ability to handle missing data. In real-world scenarios, it is common for users to have sparse rating patterns, where they have only rated a small fraction of available items. Pearson correlation can effectively handle this by disregarding missing values and focusing on the available data points. This makes it a reliable and practical method for recommending items to users with incomplete rating histories.
Another advantage of using Pearson correlation is its ability to handle non-linear relationships between variables. While it primarily measures linear associations, it can still capture certain non-linear patterns in the data. This makes it more robust than other metrics like cosine similarity, which solely considers similarities in directions between variables. By accommodating non-linear relationships, Pearson correlation can provide more accurate recommendations, ensuring that users receive suggestions that align with their actual preferences.
Furthermore, Pearson correlation takes into account the mean and standard deviation of the ratings given by users. This additional normalization step helps in removing the overall rating bias that may exist across different users. This allows the recommendation engine to focus on the relative rating patterns, rather than the absolute values, resulting in more personalized and relevant recommendations.
Lastly, Pearson correlation is computationally efficient. It can handle large datasets with a reasonable amount of processing power and time. This scalability makes it highly suitable for real-time recommendation systems, where recommendations need to be generated quickly based on the user's current behavior.
In conclusion, Pearson correlation is a crucial component of product recommendation engines. It can handle missing data, capture non-linear relationships, account for rating biases, and perform efficiently with large datasets. By leveraging these advantages, recommendation engines can deliver accurate and personalized recommendations, enhancing the user experience and driving customer satisfaction.
Understanding Collaborative Filtering
Collaborative filtering is a widely used technique in recommendation systems that helps users discover items of interest based on the preferences of similar users. It leverages the concept of Pearson correlation to establish the degree of similarity between users, enabling accurate predictions of item ratings or recommendations. By understanding collaborative filtering and the role of Pearson correlation, businesses can provide personalized and relevant recommendations to their users, leading to enhanced user satisfaction and improved conversion rates.
Pearson correlation is a statistical measure that quantifies the strength and direction of the linear relationship between two variables. In the context of collaborative filtering, these variables represent the ratings or preferences given by users to different items. The Pearson correlation coefficient ranges from -1 to 1. A value close to 1 indicates a strong positive correlation, implying that users have similar preferences. Conversely, a value close to -1 suggests a strong negative correlation, meaning users have dissimilar preferences. A value close to 0 signifies no correlation.
To calculate the similarity between users using Pearson correlation, the ratings given by both users to a set of items are compared. The correlation coefficient is then computed by dividing the covariance of the ratings by the product of their standard deviations. This coefficient serves as a measure of similarity, enabling the identification of users with similar preferences.
In collaborative filtering, the similarity between users is used to predict their unknown ratings for items they have not yet rated. The technique takes into account the ratings of similar users and calculates a weighted average to estimate a user's rating for a specific item. By utilizing the preferences of similar users, collaborative filtering provides personalized recommendations that are tailored to individual users' specific tastes and preferences.
Understanding collaborative filtering and how Pearson correlation is employed within it enables businesses to build effective recommendation systems. By accurately predicting user preferences and providing relevant recommendations, businesses can create a more personalized experience for their users, ultimately leading to higher user engagement and satisfaction. Additionally, the utilization of collaborative filtering can drive increased conversions and revenue as users discover new items that align with their interests.
In summary, collaborative filtering, powered by Pearson correlation, is a powerful technique for building recommendation systems that offer users personalized and relevant suggestions. By leveraging the similarities between users' preferences, businesses can provide enhanced user experiences, driving engagement and ultimately boosting their bottom line.
How automation enhances AI integration in product recommendations
Automated technologies have significantly revolutionized the field of artificial intelligence (AI) integration in product recommendations, making it more accurate, efficient, and seamless. One such technique that has gained immense popularity is Pearson correlation.
Pearson correlation is a statistical measure that evaluates the linear relationship between two variables. In the context of product recommendations, it enables automated systems to determine the correlation between user preferences and available products, thereby facilitating personalized suggestions.
Automation plays a crucial role in enhancing AI integration in product recommendations using Pearson correlation. Here's how:
1. Data collection and preprocessing
Automation simplifies the data collection and preprocessing stages, enabling AI systems to analyze vast amounts of user and product data effortlessly. Automated tools efficiently fetch data from multiple sources, such as user behavior, purchase history, and demographics, and transform it into a format suitable for analysis.
2. Scalability and real-time updates
By automating the process, AI integration becomes readily scalable to handle large volumes of data. This scalability ensures that the product recommendation engine can adapt to increasing user demands without compromising performance. Additionally, automation allows for real-time updates, ensuring that recommendations are continuously refined based on the latest user and product data.
3. Efficient correlation analysis
Pearson correlation requires computing pairwise correlations between user preferences and product attributes. Automation optimizes this process by utilizing parallel computing and distributed systems. These techniques enable high-speed computation of correlations, reducing the time required for accurate recommendations. Moreover, automation eliminates human errors and ensures consistency in the correlation analysis.
4. Enhanced personalization
Automation enables AI systems to provide highly personalized recommendations by leveraging Pearson correlation. By continuously analyzing user behavior and product attributes, automation ensures that the recommendations align closely with user preferences. This personalized approach not only enhances the user experience but also increases the effectiveness of product recommendations.
In conclusion, automation plays a vital role in enhancing AI integration in product recommendations through the utilization of Pearson correlation. By automating data collection, preprocessing, and correlation analysis, it enables efficient, scalable, and personalized recommendations. Leveraging automation in AI integration ensures that the process is seamless, accurate, and adaptable to evolving user preferences and market dynamics.
Conclusion
In conclusion, the Pearson correlation is a powerful statistical tool that measures the strength and direction of the linear relationship between two variables. It provides valuable insights into the connection between variables and is widely used in various fields, including social sciences, finance, and healthcare.
One key point to consider is the interpretation of the correlation coefficient. A value close to +1 indicates a strong positive correlation, meaning that as one variable increases, the other variable also tends to increase. On the other hand, a value close to -1 suggests a strong negative correlation, implying that as one variable increases, the other variable tends to decrease. A correlation coefficient near 0 indicates a weak or no linear relationship.
Another important aspect to consider is the significance of the correlation coefficient. It is important to determine whether the observed correlation is statistically significant or simply due to chance. This can be done by conducting hypothesis tests and calculating p-values, which provide a measure of the likelihood of obtaining the observed correlation coefficient by chance.
Furthermore, the Pearson correlation assumes that the relationship between the variables is linear. If the relationship is nonlinear, the correlation coefficient may not accurately capture the true association between the variables. In such cases, alternative correlation measures, such as Spearman's rank correlation or Kendall's tau, may be more appropriate.
In practical applications, the Pearson correlation can be used to guide decision-making and make predictions. For example, in finance, a positive correlation between two stocks suggests that they tend to move in the same direction, which can help investors diversify their portfolios. In healthcare, the Pearson correlation can be used to examine the relationship between risk factors and disease prevalence, enabling researchers to identify potential interventions.
Overall, understanding and effectively applying the Pearson correlation can provide valuable insights into the relationships between variables. By embracing this statistical tool, researchers, analysts, and decision-makers can make more informed decisions and drive meaningful improvements in their respective fields.
Enhance your data analysis capabilities and gain deeper insights by incorporating the Pearson correlation into your statistical toolkit. Start exploring and leveraging this powerful technique today!