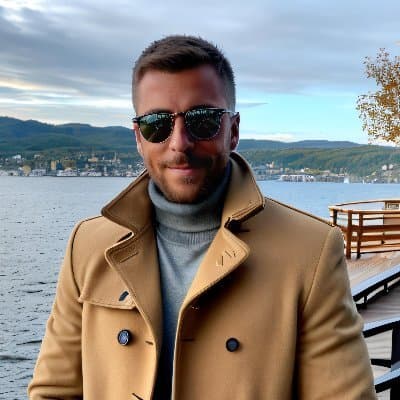
- Sep 4, 2023
- 2 min read
Unlocking Business Success with Time-Series Analysis: A Guide to eCommerce Solutions
Introduction to Time-series Analysis
Time-series analysis is a widely used statistical technique to analyze and predict patterns in sequential data. It allows us to extract valuable insights from data that are collected at regular intervals, revealing trends, seasonality, and other patterns that may not be apparent at first glance.
In today's data-driven world, time-series analysis has become increasingly important across various domains including finance, economics, weather forecasting, and even social media. By studying past and present data points, we can make informed predictions about future outcomes, helping businesses make better decisions and strategize effectively.
One key aspect of time-series analysis is its focus on analyzing data in chronological order. This sequential nature makes it particularly useful for forecasting future values based on historical observations. Time-series models can capture both short-term fluctuations and long-term trends, enabling us to understand the underlying patterns and make accurate predictions.
In this article, we will explore the fundamentals of time-series analysis, including different techniques and models that can be applied. We will discuss concepts like stationarity, autocorrelation, and lagging, providing a comprehensive understanding of the analysis process. Additionally, we will delve into popular time-series models such as ARIMA, SARIMA, and exponential smoothing methods, highlighting their strengths and applications.
Whether you are a data scientist, economist, or business analyst, mastering time-series analysis can greatly enhance your ability to make sound predictions and inform decision-making processes. So let's dive into the world of time-series analysis and unlock the valuable insights hidden within sequential data.
Understanding Time-Series Analysis
Time-series analysis is a statistical method used to analyze data points collected over a period of time. It provides valuable insights into identifying patterns, trends, and seasonal variations in data. This technique is widely used in various fields, including finance, economics, weather forecasting, and stock market predictions.
One of the fundamental concepts in time-series analysis is stationary data. Stationary data has constant mean, variance, and autocovariance over time. By ensuring stationarity, we can make accurate predictions and draw meaningful conclusions. To achieve this, we may need to transform or differencing the data.
There are several key components to time-series analysis. The first is trend analysis, which helps identify long-term patterns. Trend analysis can be linear or non-linear, providing insights into whether the data is experiencing growth or decay over time. Seasonal analysis is another crucial component, examining recurring patterns within a specific time period, such as yearly, monthly, or weekly.
Smoothing techniques, such as moving averages or exponential smoothing, are employed to reduce noise and reveal underlying trends more clearly. These techniques involve averaging data points within a defined time window to generate a smoothed series.
Time-series models are widely used to predict future values based on historical data. Popular models include Autoregressive Integrated Moving Average (ARIMA) and Seasonal ARIMA (SARIMA). These models take into account both the temporal dependence and seasonal patterns to make accurate forecasts.
In conclusion, time-series analysis is a powerful statistical method that allows us to uncover hidden patterns within time-dependent datasets. By understanding the different components and techniques involved, we can gain valuable insights, make predictions, and improve decision-making processes across various industries.
Applications of Time-Series Analysis in eCommerce Solutions
When it comes to eCommerce, businesses strive to anticipate customer behavior and optimize their operations for maximum efficiency. Time-series analysis, a statistical technique, has proven to be invaluable in achieving these goals. By analyzing historical data, businesses can uncover patterns, trends, and seasonality that can guide decision-making.
One of the primary applications of time-series analysis in eCommerce is demand forecasting. By examining past sales data and considering influencing factors like promotions, seasons, and economic conditions, businesses can accurately predict future demand. This aids in inventory management, ensuring that the right products are available to meet customer needs while minimizing excess stock.
Another crucial aspect of eCommerce is anomaly detection. Time-series analysis can help identify unusual patterns in data, such as sudden spikes or dips in sales. This can be indicative of fraud, system errors, or external events. By spotting anomalies early, businesses can take prompt action to rectify issues and mitigate potential losses.
Personalization is a key driver of eCommerce success, and time-series analysis plays a role here too. By analyzing user behavior over time, businesses can identify individual preferences and tailor recommendations and marketing campaigns accordingly. This enhances the customer experience, driving engagement, loyalty, and ultimately, sales.
Time-series analysis is also used in pricing optimization. Dynamic pricing strategies, which adjust prices based on market conditions, can benefit from analyzing historical data. By examining past pricing patterns and their impact on sales volume and revenue, businesses can optimize pricing strategies to maximize profits.
Furthermore, time-series analysis is employed in supply chain optimization. By studying order history, businesses can identify lead times and optimize inventory replenishment. This minimizes stockouts and reduces order processing time, leading to improved customer satisfaction and increased revenue.
In conclusion, time-series analysis in eCommerce offers numerous valuable applications. From demand forecasting and anomaly detection to personalization, pricing optimization, and supply chain management, businesses can leverage this technique to improve decision-making, operational efficiency, and customer satisfaction. By embracing time-series analysis, eCommerce businesses gain a competitive edge in a rapidly evolving digital landscape.
Improving Inventory Management Systems with Time-Series Analysis
Time-series analysis is a powerful statistical technique that enables businesses to make informed decisions based on historical data trends. When applied to inventory management, it can vastly improve effectiveness and efficiency. Here, we explore how time-series analysis can optimize inventory management systems and transform businesses.
Accurate Demand Forecasting
One of the key components of successful inventory management is accurate demand forecasting. By analyzing historical sales data using time-series models, businesses can uncover patterns, seasonality, and trends that help predict future demand. This enables them to optimize inventory levels, reduce stockouts, and avoid overstocking. Time-series analysis methods such as exponential smoothing, ARIMA models, or state space models can capture complex demand patterns and generate reliable forecasts.
Optimized Order Planning
Analyzing time-series data can provide businesses with valuable insights into lead times, order quantities, and reorder points. By identifying lead time variations and supplier performance patterns, businesses can adjust their reorder points and place orders more strategically. Additionally, time-series analysis allows for optimal order quantity determination by considering factors like carrying costs and economic order quantity. This not only minimizes costs but also ensures smooth operations.
Effective Seasonal Inventory Management
Seasonal products often pose inventory management challenges due to fluctuating demand. Time-series analysis helps businesses handle seasonal inventory management more effectively. By quantifying seasonality and understanding demand patterns during different periods, businesses can optimize inventory levels, promotional strategies, and pricing. This ensures sufficient stock during peak seasons while minimizing stock holdings during quieter times, avoiding unnecessary costs.
Mitigated Forecast Errors
Forecast errors can lead to costly consequences, such as excessive stock or stockouts. Time-series analysis provides businesses with means to quantify and mitigate these errors. By monitoring forecast accuracy and analyzing residuals, businesses can identify and rectify the root causes of errors. This enables continuous improvement and enhances the accuracy of future forecasts, leading to better inventory planning and reduced costs.
Continuous Performance Evaluation
Implementing time-series analysis in inventory management allows businesses to continuously evaluate their performance and identify areas for improvement. By comparing forecasted demand with actual sales data, businesses can measure forecast accuracy, track trends, and adjust their models accordingly. Ongoing evaluation enables proactive decision-making and optimization, ensuring inventory management systems remain efficient and adaptive to evolving business needs.
In conclusion, time-series analysis offers immense potential for improving inventory management systems. Accurate demand forecasting, optimized order planning, effective seasonal inventory management, and mitigated forecast errors are some of the key benefits it provides. By embracing this technique, businesses can optimize inventory levels, reduce costs, and enhance their overall supply chain operations.
Leveraging Time-Series Analysis for Accurate Demand Forecasting
Accurately predicting demand is crucial for businesses to optimize inventory planning, production schedules, and resource allocation. Time-series analysis, a statistical technique, provides a powerful framework to analyze and forecast future patterns based on historical data.
One widely used method in time-series analysis is the Autoregressive Integrated Moving Average (ARIMA) model. ARIMA identifies trends and patterns in a dataset by considering three key components: autoregression (AR), differencing (I), and moving average (MA). By analyzing historical patterns, ARIMA models can forecast future demand with remarkable precision.
Another valuable technique for demand forecasting is Seasonal Decomposition of Time Series (STL). STL decomposes a time-series into three components: trend, seasonality, and residuals. This decomposition allows businesses to understand the underlying patterns and make more informed decisions. By isolating seasonal effects, businesses can adjust inventory levels, marketing campaigns, and production schedules accordingly.
Additionally, Exponential Smoothing (ES) models are widely employed for demand forecasting. These models assign exponentially decreasing weights to older observations, giving more importance to recent data. The simplicity of the ES models allows them to handle large datasets efficiently. They can capture trends, seasonality, and irregular variations, making them suitable for short-term and long-term forecasts.
Furthermore, modern advancements like Artificial Neural Networks (ANNs) have gained popularity in demand forecasting. ANNs can analyze complex patterns and nonlinear relationships in a time-series dataset. By training on historical data, ANNs can identify hidden relationships and capture intricate demand dynamics, resulting in accurate predictions.
Implementing time-series analysis techniques can enhance demand forecasting accuracy and help businesses make data-driven decisions. However, before applying these models, it is essential to handle outliers, missing values, and stationarity issues in the dataset to ensure reliable results. Moreover, regular model validation and retraining with updated data ensure ongoing accuracy.
In conclusion, leveraging time-series analysis techniques like ARIMA, STL, ES, and ANNs can provide businesses with precise demand forecasts. Accurate demand forecasting enables more effective resource allocation, inventory management, and production planning, minimizing costs and maximizing customer satisfaction. By harnessing the power of time-series analysis, businesses can stay ahead in today's highly competitive markets.
Conclusion
In conclusion, time-series analysis is a valuable technique for understanding and predicting trends in data that changes over time. By analyzing the temporal patterns and identifying underlying components, such as trend, seasonality, and noise, it provides valuable insights for businesses, researchers, and analysts alike.
Throughout this article, we have covered the fundamentals of time-series analysis. We began by explaining the concept of time-series data and the importance of its characteristics. We then explored various methods and models used in time-series analysis, including smoothing techniques like moving averages, exponential smoothing, and ARIMA models. Additionally, we discussed advanced techniques like seasonal decomposition, state space models, and forecasting.
The applications of time-series analysis are vast and varied. It finds practical use in finance, economics, weather forecasting, sales forecasting, stock market analysis, and many other domains. By utilizing time-series analysis, organizations can make informed decisions, detect anomalies, identify patterns, and develop accurate forecasts.
To leverage the power of time-series analysis, it is crucial to have access to reliable, high-quality data. Additionally, it is important to continuously update and refine models as new data becomes available. By investing in the necessary tools and resources, businesses can effectively harness the predictive capabilities of time-series analysis to gain a competitive advantage.
In conclusion, time-series analysis presents immense opportunities for those who recognize its potential. By applying this technique to real-world problems, businesses can optimize their operations, minimize risk, and improve overall decision-making. Let's embrace the power of time-series analysis and elevate our understanding and prediction capabilities to drive success in an ever-changing world.